
This is Part 2 of our deep-dive into AI music-making. In Part 1, we learned what AI is; examined the challenges of applying AI technology to music-making; and explored uses of AI such as MIDI generation, timbral transfer, and analog modeling.Â
In this second part, weâll take a broader look at AIâs impact on music-making. Weâll explore vocal deepfakes and speculate on the possibility of a ChatGPT for music. And weâll examine some of the profound questions raised by AI, concerning creativity, originality, and what it means to be a musician.
Entering the deepfake era
The human voice has a unique position in our culture. No sound comes closer to expressing an authentic, unique self. Even when processed with effects like autotune, a voice is closely tied to a person - usually one person in particular. The singing or speaking voice is the ultimate sonic stamp of personhood. At least, it was.
What if we could have a voice without a human? Tools like Yamahaâs Vocaloid have long offered to synthesize voices from scratch. But the robotic results only really worked in situations where the artificiality was the point (such as virtual popstars like Hatsune Miku). AI tools are better at this task. With deep learning, itâs possible to generate voices that are so lifelike that they can trick the listener into âhearingâ a person.
Take a plug-in like Dreamtonicsâ Synthesizer V. You input the MIDI notes and lyrics, and select a voice bank with the desired characteristics (like Natalie, âa feminine English database with a voice that presents a soft and clear upper range as well as rich and expressive vocals on lower notes.â) And out comes a voice. The results are variable, but at their best could easily fool a casual listener. Indeed, they regularly do. Last year, Chinese company Tencent Music Entertainment revealed that it had released over 1000 songs featuring AI-generated voices.Â
The implications for the more commercial end of the music industry are profound. (Major labels must be intrigued by the idea of pop music without temperamental popstars.) But while voice synthesis using generic voice banks has many functional uses, it probably wonât replace human popstars any time soon. When we listen to our favorite singer or rapper, weâre enjoying that voice in particular: its timbre and grain, and its connection to a person who represents something we care about. Anonymous synthesized voices canât compete with the aura of an artist.Â
But what if AI could imitate the voices we love? This April, the internet lost its mind over a new collaboration between Drake and The Weeknd, âHeart On My Sleeve.â So far, so normal - except the track was entirely fake, having been created using AI voice cloning technology by an artist calling themselves Ghostwriter. A few weeks previously, AI entrepreneur Roberto Nickson caused a similar stir when he used an AI tool to transform his voice into Kanye West.Â
AI voice cloning is a cousin of the timbral transfer technology we explored in part 1. But where timbral transfer plug-ins like Neutone sound like a tech in its infancy, voice cloning tools are getting shockingly good. This is true for speech as well as singing. Voice cloning AI company ElevenLabs caused consternation last year when they transformed Leonardo DiCaprioâs voice into Bill Gates, Joe Rogan, and others. They soon reported that pranksters were using their tool to make celebrities say offensive and inflammatory things.
We are entering a new era of âdeepfakes.â Just as image generation tool Midjourney can convince us that the Pope wears Balenciaga, so too must we approach every recorded voice we hear with skepticism. But for electronic producers, this could present an opportunity. Sampling is a bedrock of electronic music, and sampled voices - whether full acapellas, sliced up phrases, or bursts of spoken word - are threaded through many dance music genres. This practice emerged from the more permissive sampling culture of the â80s and early â90s, but these days it can fall foul of litigious rights holders. What if AI allowed producers to âsampleâ their favorite vocalists without breaching copyright at all?
French club producer Jaymie Silk has long used sampled voices from movies or speeches in his music. On his 2021 Track âA President Is Just A Gangster With A Nuclear Weapon,â he got an iPadâs robotic text-to-speech function to recite the titular phrase. In late 2022, looking to push the idea further, he stumbled across an AI tool - he canât remember which, but it couldâve been FakeYou - that offered text-to-speech with the voices of famous rappers and singers. He immediately saw its potential, and wanted to be the first to use the tool in a club context. (He was right to rush; a few months later, David Guetta âsampledâ Eminem in the same way).
The result was Rub Music Vol. 1, an EP of pumping club tracks featuring vocal âsamplesâ from artists like The Weeknd, Kendrick Lamar, and Tupac. The lyrics - written by Silk himself, of course - hint at the EPâs concerns: in âIllusions,â Tupac says, âWhat is real is not real⌠Everything is an illusion.â But while Silkâs EP expresses some deepfake angst, it also has the playful feel of a music-maker exploring an exciting new tool.
Voice cloning technology has improved since Silk made the EP. (He continued to use AI voices on his more recent release, Letâs Be Lovers). âIt was basic at the time,â he remembers. âYou couldn't modify or apply settings. Youâd type something in, and you could be lucky.â The sound quality was also not ideal. In some cases - as in The Weekndâs voice on âArtificial Realnessâ - extensive post-processing couldnât remove the sibilant artifacts.
More recent voice cloning tech sounds better. Itâs easy to imagine a tool combining the celebrity cast of FakeYou with the features of a voice synth like Synthesizer V. The result would be a mighty âsamplingâ toolbox, allowing you to have any popstar imaginable sing or rap words of your choosing.Â
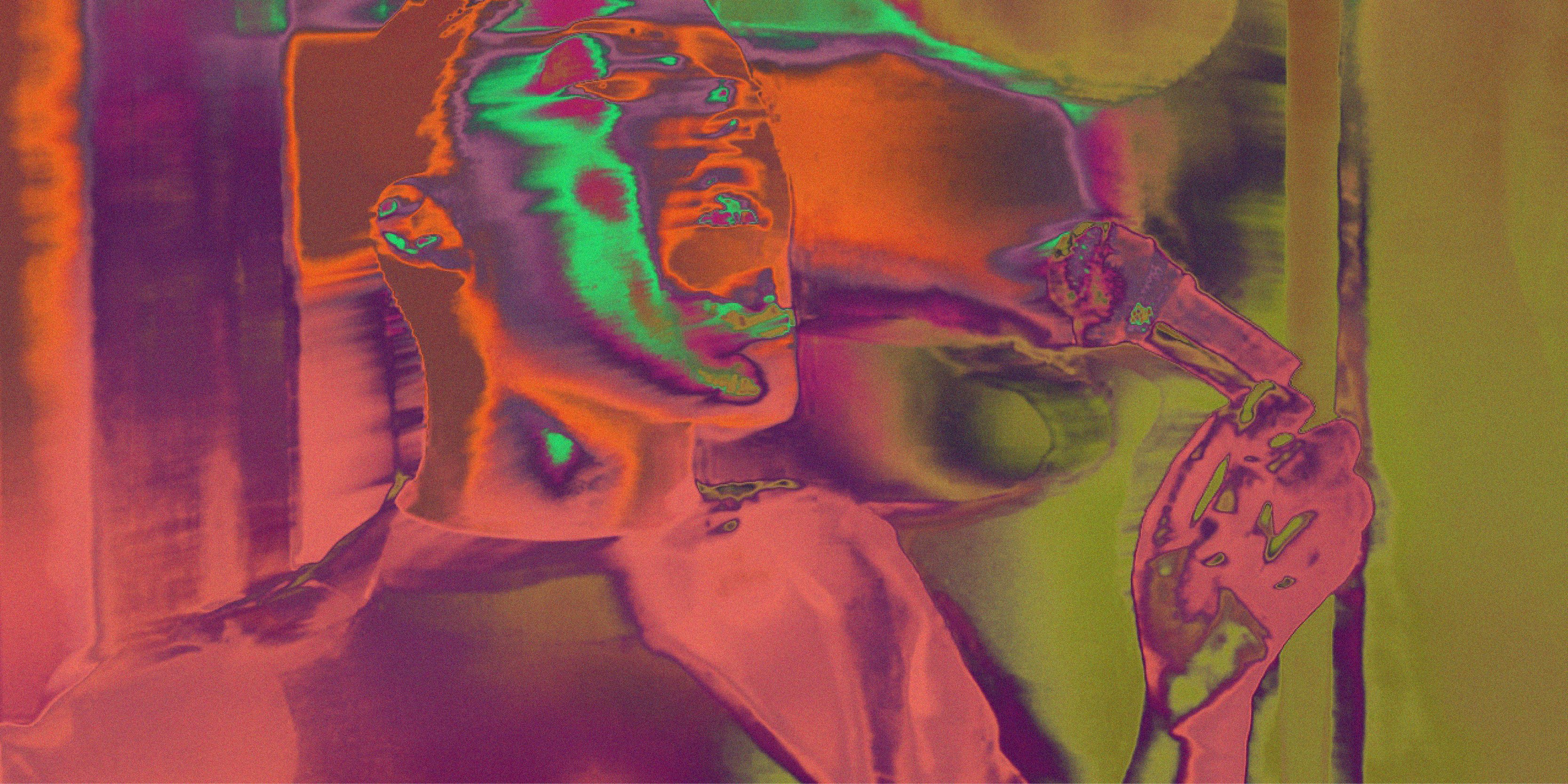
Who owns your voice?
But is this actually legal - or, for that matter, ethical? As weâve discussed, a vocalistâs voice is the stamp of their personhood, and the main tool of their self-expression. Having this voice replicated a thousand times over could spell financial and creative ruin for many artists. Shouldnât a vocalist get to decide who uses their sonic likeness?
Social context makes this question more pressing. Discussing the potential downsides of AI tools, Silk mentions the term âdigital blackface,â which was leveled at Roberto Nickson for his Kanye video. Critics of Nickson, who is white, suggested that such tools provide a new way for white people to play with and profit off of black artistsâ identities: a toxic dynamic at least as old as popular music.Â
If we consider voice cloning as a new form of sampling, then the dynamic that is emerging calls to mind injustices at the root of sampling culture. World-famous samples that have powered dance music for decades - such as the Amen and Think breakbeats - were performed by musicians who were never properly remunerated for the impact of their work. Itâs easy to imagine AI voice tech having a similar exploitative dimension.
Some people saw this coming a long way off. Following her experiments with timbre transfer on 2019âs PROTO (discussed in Part 1), the musician Holly Herndon launched Holly+ in 2021. At the heart of the project is a high quality AI voice model of Herndonâs own voice: her âdigital twin.â Users can interact with this model via a website, by uploading audio and receiving a download of the music âsung backâ in Herndonâs âdistinctive processed voice.â Itâs like Jlinâs beats being sung on PROTO - but accessible to all, and in much higher quality.
As her statement on Holly+ explains, Herndon launched the project to address questions around âvoice ownership,â and to anticipate what she sees as a trend of the future: artists taking control of their own âdigital likenessâ by offering high quality models of their voice for public use. This way, the artist can retain control over their voice, and perhaps profit from it. (Using Holly+ is free, but profits from any commercial use of the model go to a DAO, which democratically decides what to do with the money.)
According to Herndon, the voice cloning offered by tools like FakeYou may in fact violate copyright law - at least in the US. Providing context around âvoice model rights,â Herndon cites legal cases back to the â80s in which public figures were protected âagainst artists or brands commercially appropriating their vocal likeness.â These precedents âsuggest that public figures will retain exclusive rights to the exploitation of their vocal likeness for commercial purposes.â And indeed, UMG had the Drake x The Weeknd song taken down within a few days, arguing that music made with AI tools trained on their artistsâ music violates copyright law.
A legal and ethical infrastructure needs to be built around these fast-developing tools. But, as with file-sharing in the 2000s, lawmaking may not return the genie to its bottle. Vocalists could find themselves competing - for attention, and perhaps for work - with their own digital likeness. In fact, itâs not only singers who feel this fear of replacement. Cheap or free-to-use image generation tools have become a tempting option for companies reluctant to pay a human illustrator. ChatGPT, meanwhile, fills professional copywriters with dread. The question is spreading through the creative industries and other white collar professions: Will AI take my job?
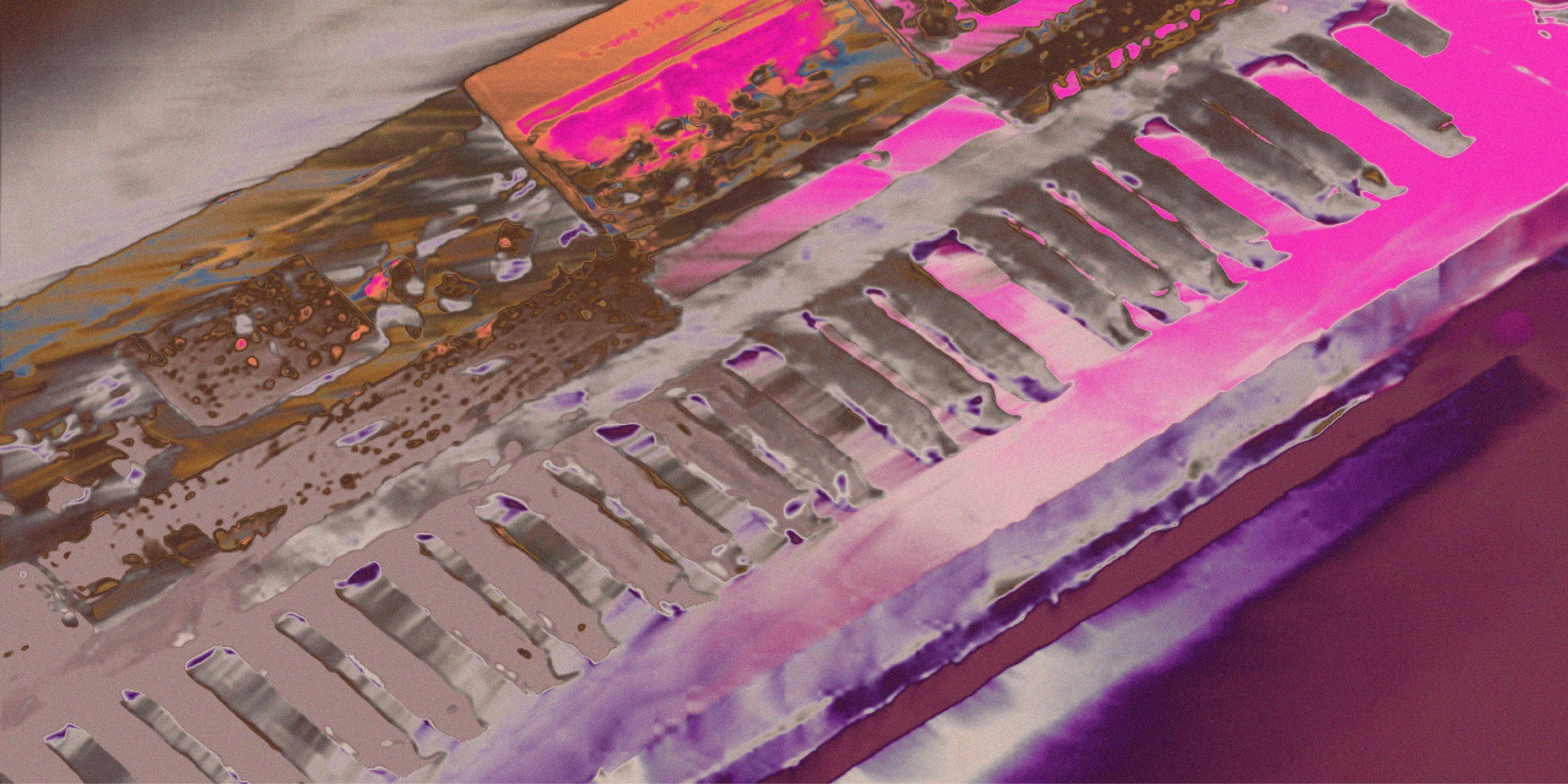
Automated composers
This brings us back to a question we touched on in Part 1. Tools such as ChatGPT and Stable Diffusion compete with human creators because of their sophistication and wide availability. An equivalent tool - powerful, good quality, and widely accessible - doesnât yet exist for music. (We explored the reasons why in Part 1). But will it soon?
The answer from the specialists is a firm yes. Mat Dryhurst from Spawning mentions several organizations that are working on such a model. One is Google, whose MusicLM was introduced to the world at the beginning of this year, but isnât yet publicly available. (Google started opening MusicLM to small groups of testers in May.) Another is HarmonAI, a music-focussed organization affiliated with Stability AI, the creators of the Stable Diffusion text-to-image model. HarmonAI involves The Dadabots, who have said that we can expect a tool from the organization âthis year.â
To understand how such a tool might change the music-making landscape, we can start by looking at the less sophisticated AI music generators that already exist. While a âgeneralâ music model remains elusive for now, AI is already creating music in more limited contexts. In contrast to the tools explored in Part 1, these AI technologies arenât typically designed to support existing music-making processes. Instead, they offer to remove the need for a skilled music-maker entirely - at least in certain situations.
Commercial composition is one such situation. Our world is lubricated by multimedia content, and there is an inexhaustible demand for soundtracks to adverts, podcasts, and social media posts. The makers of this content have a few options. They can commission a new composition at significant cost, or license a track from their favorite artist, probably for a hefty sync fee. Or they can source a cheaper soundtrack via a music library - the music equivalent of Shutterstock. But what if none of the music they can afford quite fits their needs? Or if their budget is tiny?
Here, AI products such as AIVA step in. AIVA began life in 2016 as an AI model trained to compose classical and symphonic music. (It was the first âvirtualâ composer to be recognized as such by a music rights society). The technology was made commercially available in 2019, and now presents itself as a âcreative assistant,â promising to help you come up with âcompelling themes for your projects faster than ever before.â
The process of generating a track is simple. The basic version is free to use. You hit âcreate a trackâ and start narrowing down your options. 12 preset styles, ranging from â20th Century Cinematicâ to âHip Hop,â set the frame in which the AI should work. You then pick parameters from a dropdown menu - key, tempo, instrumentation, and duration.
I chose a fast-paced âFantasyâ track performed by solo strings, and got 3 minutes of arpeggios with some disjointed melodic turns. It wouldnât convince a close listener, but could work fine mixed at background level in a low-budget project. If I wanted, I could tweak the generation further in the MIDI-based editor mode. (The MIDI file can also be downloaded for further use).  Â
AIVA can be effective with less sophisticated AI technology because it works in a tightly defined frame. Its preset styles and dropdown menus are a far cry from the anything-goes realm of natural text prompts. But when itâs formulaic, functional music you need, this method could work fairly well. Â
Should professional composers be worried? The answer probably echoes our discussion of mixing automation in Part 1. AI may soon be able to handle formulaic briefs where inspiration isnât required (or desired). Higher-stakes projects will probably still benefit from a humanâs creative vision. Perhaps a two tier system will emerge, with a human composer becoming a mark of high quality media. In other words, humans may become the premium choice.
This, at least, is one possible outcome suggested by generative AI composers. Other tools lead to a different conclusion. What if AI makes us all musicians?
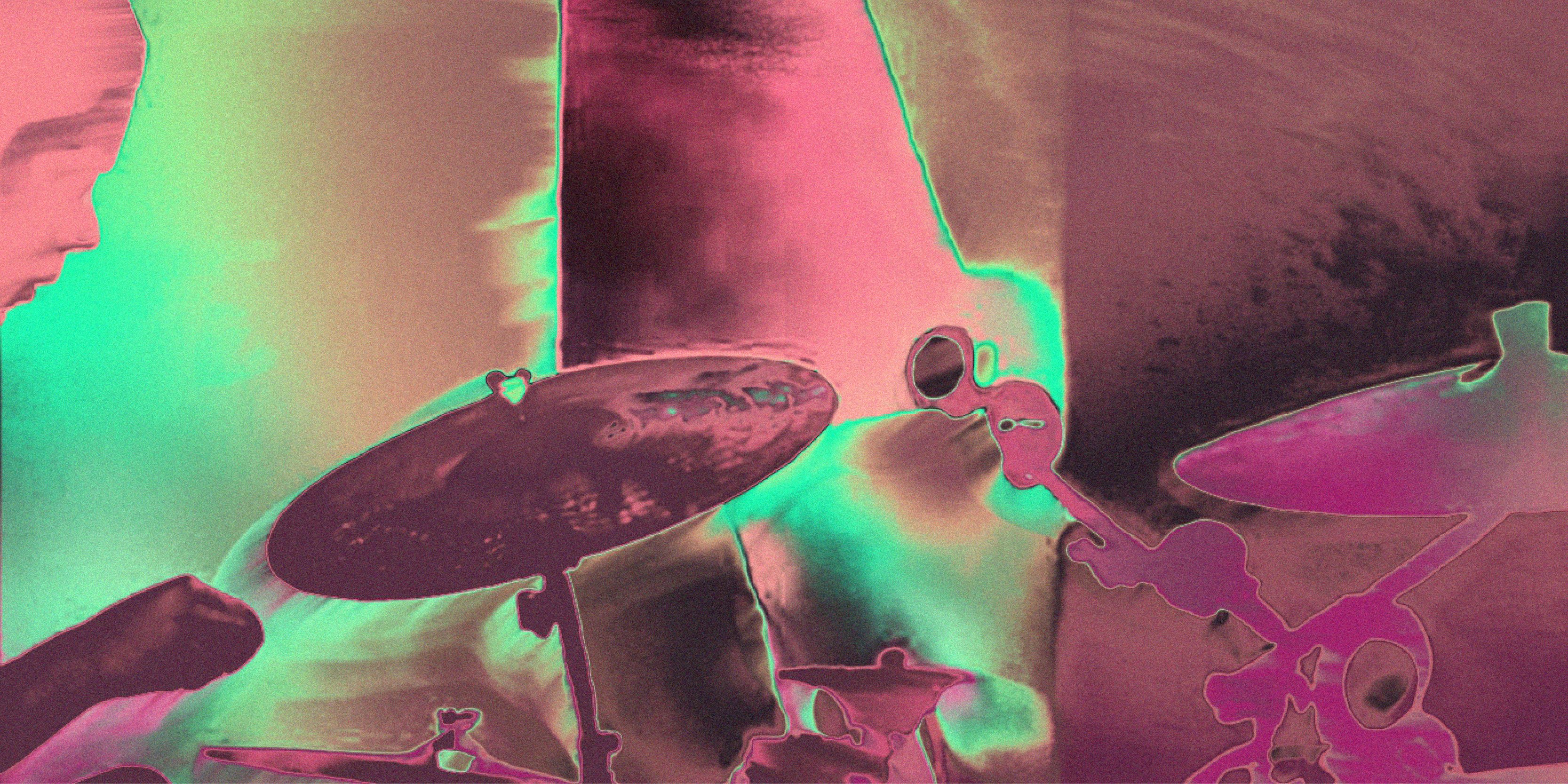
Everyoneâs a music-maker
Boomy is an AI-based platform that invites you to âmake original songs in seconds, even if you've never made music before.â It works similarly to AIVA. You navigate through dropdown menus of styles and sub-styles, and the AI generates a composition to your spec. You can then tweak the results with a simple editing suite.Â
Like AIVA, the tool gives you creative control within an extremely limited frame; and like AIVA, the results arenât guaranteed to sound great. This hasnât put off its user base. According to Boomy, the tool has been used to generate some 13 million songs, many of which have been uploaded to Spotify through the site and monetized by their creators.Â
Tools like AIVA and Boomy are no more than a glimpse of what might be coming. So far, their claim to supplant skilled music-makers is shaky even within the limited contexts that they address. But the rapid advances in AI in recent years should teach us not to dismiss this technology out of hand.Â
Google shared audio examples when introducing MusicLM, probably the most sophisticated text-to-music model so far presented to the public. Many of them are interesting mainly for their strangeness. (See, for example, the alien skronk prompted by the word âswingâ). But others are more convincing. One 30 second clip - âa fusion of reggaeton and electronic dance musicâ - could be the start of a pretty compelling club track.Â
âThe central challenge for music-makers will stay the same: how to break through the noise and reach an audience that cares.â
More recent examples shared online by GoogleLMâs testers demonstrate the same mix of the promising and the downright bizarre. But we should keep in mind the rapid progress of text-to-image tools over the past year, from smudgy sketches to hi-resolution deepfakes. Why shouldnât it be similar for music? If this is where the technology is now, where might it be in a few years? Will it be possible for anyone to generate a decent-enough techno track in a few seconds?
âWe live in the era of democratization of technology,â says Jaymie Silk. But this era started before AI came along. For decades, advances in technology have enabled more and more people to make music and share it with the world. Itâs common to hear complaints that there is âtoo muchâ music being released. This doesnât stop us from celebrating the artists who bring beauty and meaning into our lives.Â
Whether those artists can make a living is a different issue. The economics of music-making were tough long before AI came along, and AI could make them worse. The question of how musicians might make a living in an AI-powered age requires serious thought. But placing music-making into more peopleâs hands doesnât mean there will no longer be special or profound music.
âWhen it becomes trivial for anyone to produce media to a certain level of sophistication, that just shifts our perception of what is banal, and background,â says Dryhurst. âIt was once very laborious and technical to produce electronic music. Now anyone can buy a sample pack and some software, follow a tutorial on YouTube, and make something ok. That is not a bad thing, and that is often how people begin to learn how to express themselves. Automating that process even further just changes our baseline expectations, and says nothing about what artists are going to create to distinguish themselves from what you can now make with a click of a button. It will still take great technical skill, or inspiration, or luck, to create something that stands out. That has always been difficult, and will remain so.â
Jaymie Silk agrees. âThere will be more shitty music, or more people doing music for fun.â But the central challenge for music-makers will stay the same: how to break through the noise and reach an audience that cares. âThis part will not change. You still have to make good music, you still have to build a community.â
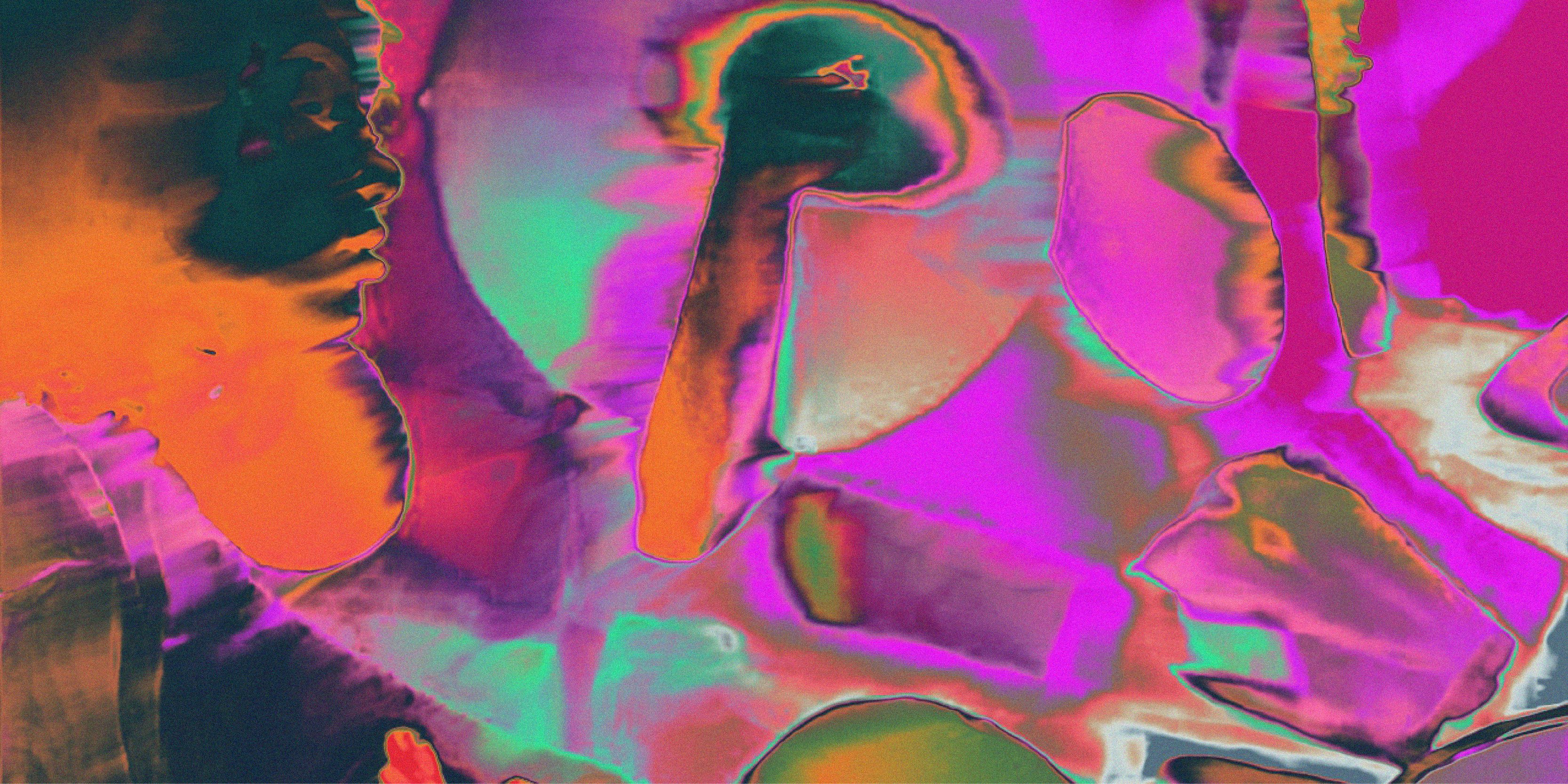
Spawning the future
Artists will use these new tools in expressive and imaginative ways, just as they have with new technologies in the past. In fact, theyâre doing it already.Â
The London-based artist patten stumbled across Riffusion late last year. He was already familiar with generative AI from his work as a graphic designer. Riffusion caught his musicianâs ear.Â
Launched towards the end of 2022, Riffusion was a hobby project that had an outsize impact. Rather than tackling text-to-music generation head-on, it piggybacks on the more successful text-to-image generation technology that already exists.Â
This works by âfine-tuningâ - a process where you train an AI model on a specific kind of content to make it better at producing that content. The musicians Seth Forsgren and Hayk Martiros fine-tuned the text-to-image model Stable Diffusion on spectrograms (visual representations of the frequencies in a sound over time). These spectrograms can then be âreadâ and turned into audio. Voila: a text-to-image model you can hear.Â
Riffusion is a lot of fun to play with. You can feed it simple text prompts - âemotional disco,â âlatent space vaporwaveâ - and it will play you an endless stream of iterating loops. You can also download your favorites. patten recognized the tool was more than just a toy. âAfter playing around with it for a short period of time, I came to realize that there was a lot that you could do with it. So I started pushing it and trying to see what I could get out of it.â
patten gathered material in a sleepless day-and-a-half binge of prompting and downloading. Later, he went back through what heâd collected, stitching the interesting parts into âfragmentary cloud-like pieces of music.â These pieces of music became Mirage FM, which patten claims is âthe first album fully made from text-to-audio AI samples.âÂ
Itâs a beautiful, dreamlike record that doesnât sound like anything else - though it flickers with hints of familiar styles. The content was entirely generated using Riffusion, but fans of patten will recognize his trademark aesthetic. A lot of the creativity, he says, came in the way he stitched the audio together. âOften it was these really tiny fragments that were spliced together into musical phrases and loops. I suppose this [album] was really about the edit as compositional expression.âÂ
Dryhurst thinks that an approach like pattenâs will soon be common among music-makers. âPeople will think nothing of generating passages of music to use in productions.â
One curiosity of Mirage FM is that, for all its boundary-breaking newness, there is a nostalgic quality to the music. This is helped by the tinny, artifact-riddled audio. (The Dadabots suggest that this may be down to âphase retrievalâ issues caused by Riffusionâs spectrogram method.) patten likens this quality to cassette distortion or vinyl crackle. Itâs an evocative comparison, particularly taken alongside the albumâs tagline: âcrate-digging in latent space.â We might think of AI tools as a portal into the future. But, trained as they are on a vast corpus of existing music, theyâre also a window into our cultural past.
As with voice models, a comparison emerges between generative AI and sampling. Past generations of musicians dug through old music to find the one perfect sample; musicians of the future might search the âlatent spaceâ of an AI model for the choicest sounds. Only this time, the sounds might seem familiar, but theyâre unique on each generation, and copyright-free.
The sampling comparison has been made before. The Dadabots made their name training AI models on artists they loved. A series of free Bandcamp releases captured the output of models trained on bands like Battles and Meshuggah. They have also presented their work as YouTube live streams â like RELENTLESS DOPPELGANGER, a âNeural network generating technical death metal, via livestream 24/7 to infinity.â
(They report âa range of responsesâ from the artists trained on their models. Some are âintrigued,â while other projects - like their fusion of Britney Spears and Frank Sinatra - have been flagged for copyright infringement.)Â
One such live stream, from 2021, came with a treatise on sampling. âSampling serves an important use in music: there are sounds, feelings, messages, and reminders of history that can only be expressed through quotation.â But, the Dadabots wrote, copyright constraints limit musiciansâ freedom to sample in their work. âNeural synthesis gives some of this ability back to musicians. We can now synthesize music that quotes a particular era, without sampling any previously published recording.â
The sampling comparison isnât perfect, and some think itâs unhelpful. âYes, of course, there's this technical possibility of circumventing the economic impact of wanting to sample,â says patten. âBut I think there's huge potential for something more than that, which is less bound to the world of exchange and value, and is more about looking for forms of sonic experience that haven't existed before.â Dryhurst argues that we must âtreat AI as a new paradigmâ rather than falling back on old language and concepts. He and Herndon have coined a new term for the practice of generating AI audio for use in music: âspawning.â
But the idea of generative AI as consequenceless sampling helps us to address some of its ethical problems. As with voice models, the âcopyright-freeâ tag doesnât quite stick. Generative deep learning models are trained on data. The responses they give are based on patterns theyâve learned from this data. A text-to-image model like Stable Diffusion is trained on huge numbers of images, so that it can learn what makes a pleasing or accurate image - and produce one for us on demand. But where do these images come from?Â
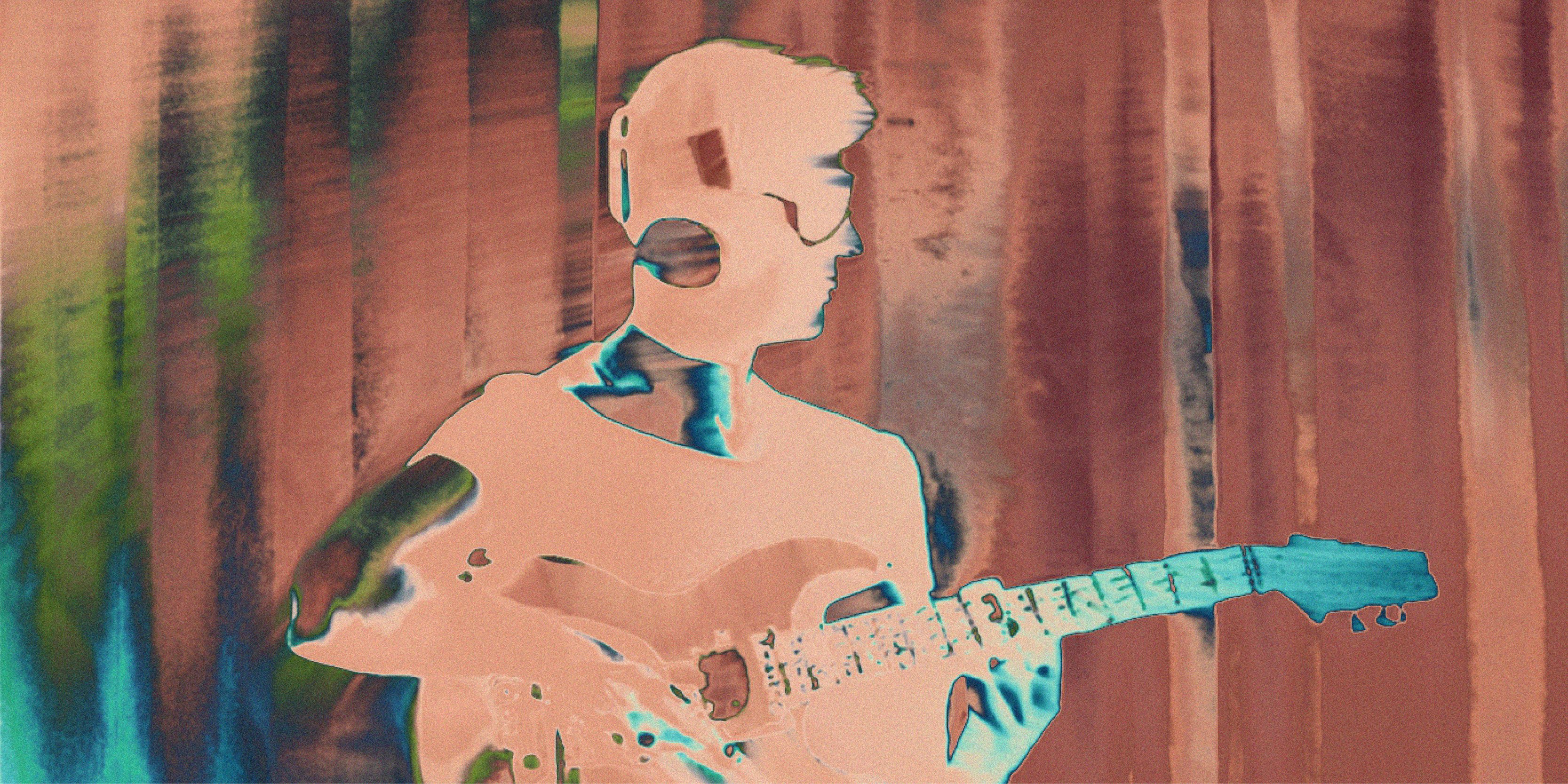
Copyright, ethics and originality
Stable Diffusion is trained on the LAION-5B image set, a massive trove of images scraped from the web. The images in LAION-5B are publicly available. But that doesnât mean the creators of those images consented to their use in training AI models. Countless images from art sites such as Deviant Art have been used to train text-to-image models; thatâs why the models are so good at generating illustration-like images in a style that we recognize.Â
Many of these artworks - and other images in datasets like LAION-5B - are copyrighted. According to current copyright law in the US and the EU, the inclusion of these artworks in a data set is allowed so long as itâs not being used for commercial purposes. But generative AI is a hugely profitable commercial enterprise - and the presence of these artworks in data sets is key to the technologyâs appeal.Â
The ethical stakes start to look similar to those involved in sampling. Generating media from a deep learning model trained on non-consenting artistsâ work isnât so different from sampling their work without permission. In both cases, the original creators canât give consent and donât get paid.
This has led to a fightback from artists and rightsholders. A series of lawsuits are underway against AI models like Stable Diffusion, with the stock photo company Getty Images among the complainants. And there is heated debate around how to make data sets more consenting.
Dryhurst and Herndon have launched the tool Have I Been Trained? which allows artists to find out whether their work has been used in major data sets, and to opt out of its use in future. There is no legal mechanism to enforce this opt-out, but the idea is already having some success. Stability AI, the company behind Stable Diffusion, have said they will honor the opt-out (which now encompasses 80 million images) in the next iteration of their model.
This war over intellectual property is being waged over images. What about audio? The music industryâs complicated ownership structures make it more resistant to the creation of consent-less data sets. In fact, some say this is partly why generative AI music models lag behind image and text: itâs harder to get the data to train them.
âThe music industry has a mind bogglingly complex structure, and the layers of organizations required to ensure enforcement of copyrights can result in cautiousness about new means of distributing music,â explain The Dadabots. âEven if an artist is excited about AI, it might not be solely up to them if the generated music can be sold. Popular artists often do not fully own their music and are sometimes not able to give permission for its use without consulting labels or publishers.â
Itâs surely no bad thing if technology has to wait a little while legal and ethical frameworks catch up. The hope is that this is reflected in forthcoming generative models. HarmonAI, for example, are taking measures to seek consenting data for their forthcoming Dance Diffusion model. Meanwhile, Have I Been Trained? intends to expand its functionality to include audio. âThe fundamentals we are putting in place will work across media type,â says Dryhurst.
Beyond the issue of consent, AIâs reliance on data sets raises questions about its scope. Critics will say that this is a fundamental limitation of AI. Trained on existing human creations, an AI model canât do anything new - just regurgitate ideas weâve already had, albeit in new combinations. In this depiction, musicians who use AI could become mere curators, reshuffling familiar ingredients in an increasingly derivative cultural soup.
On closer inspection, though, the line between âcurationâ and âcreationâ isnât so clear. âIn music there are only so many instruments that exist, there are only so many chord progressions, only so many ways to put them together,â says Christain Steinmetz. âSo a band is actually kind of curating music theory, picking the parts that they like, and packaging them into some creative material.â
patten takes the idea further. âWhen we say, â[the AI] is not doing anything new, because it's derived from existing material,â youâve got to think: What is it that we're doing in this conversation now? We're not inventing a whole system of linguistic devices to express ourselves. We're using a language that we both share and understand, with its various histories.â In this way, for patten, AI tools open up profound questions about what creativity and originality really are. âThere's this incredible opportunity to look at some age-old questions about the nature of consciousness, humanity, creativity. And to reflect on what it is that we're doing when we're doing these things - and what makes us human.â
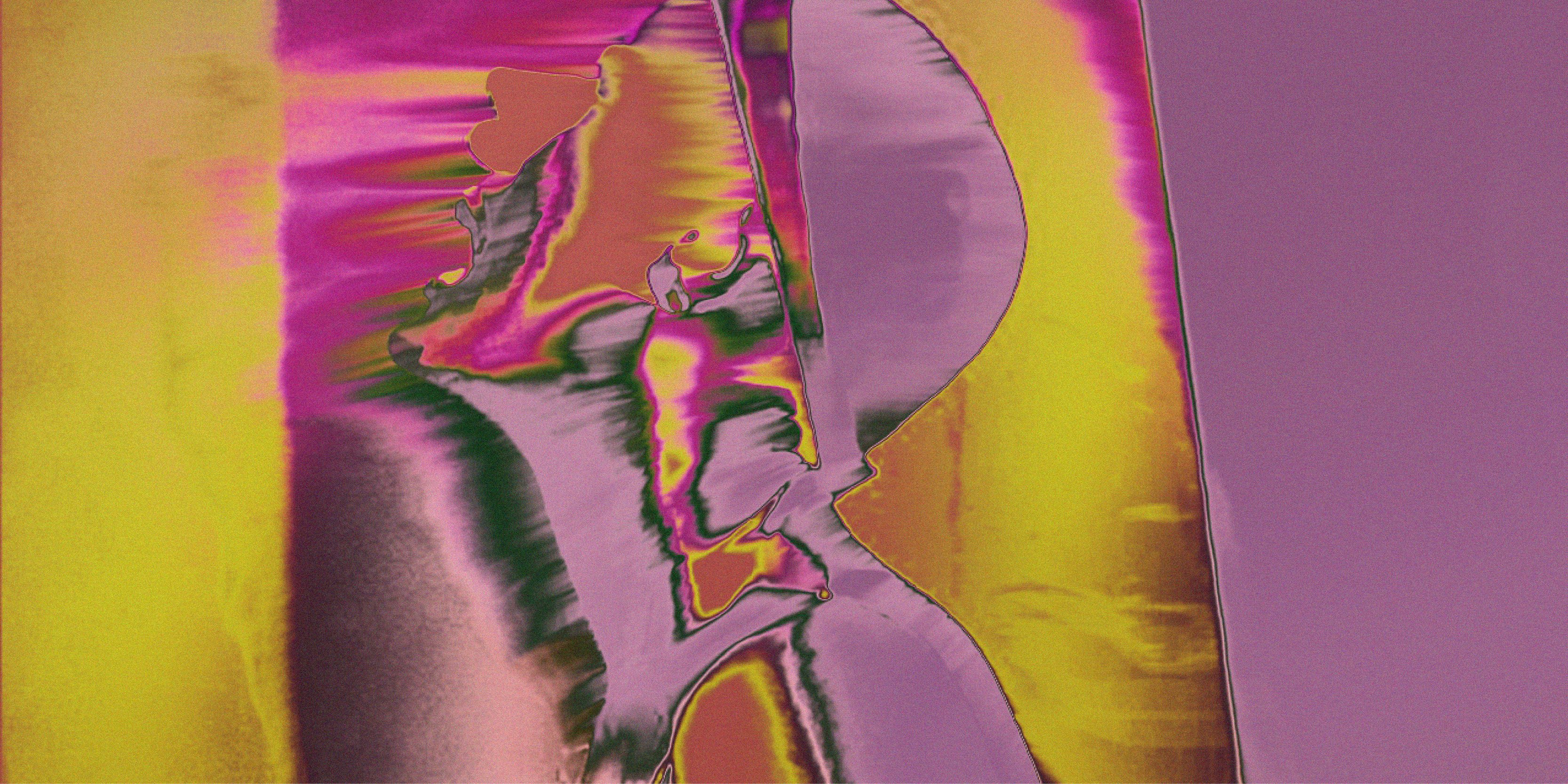
Conclusion: Money, Automation and Transitioning To The Future
In these articles weâve looked at a number of ways that AI technology could change music-making. Weâve covered a broad spectrum of activities, from taking over technical mix tasks to generating MIDI, placing famous voices in producersâ hands, and âspawningâ passages of audio and entire compositions. What unifies these different uses of AI? In each case, the AI is doing something that would have required human effort before. In other words: all of them are forms of automation.
The drive to automate has been fundamental to the last few centuries of human history. Automation means using machines to make products quicker than a human can, or getting computers to do complicated sums for us. By reducing the human effort required in a process, automation lowers costs and increases productivity. That means more money for whoever owns the automating machine.
This gives us a clue as to the driving force behind new AI technologies. It takes enormous resources (not to mention a hard-to-calculate environmental cost) to train vast deep learning models like ChatGPT and Midjourney. It is typically large tech companies that can afford to bankroll these models, and it is these companies that will reap the rewards (or so they hope).Â
AI isnât simply a story about monopolistic tech giants. There are many creative people working on AI music tools, driven by a spirit of discovery and a thirst for new sounds. But in the scheme of things, AI music is a sideshow to the main event: the automation of vast swathes of our economy.Â
History teaches us that automation is a painful process. Hard-earned skills become redundant or devalued; livelihoods are lost; cultures and communities are upended. Cushioning the impact is a political challenge, raising questions about how we organize our societies and who and what we value. Battles over the meaning and implications of AI technology are already being fought, and they will intensify in the coming years.Â
But looking at history, we can see that these upheavals have never spelled doom for musical creation itself. Such moments shift the frame of what we consider to be music, and what we consider to be a musician. Some musical traditions lose their relevance, but previously unimaginable new ones emerge. Silicon Valley didnât have techno music in mind when the microprocessor was invented. But a chain of events was set in motion that led to mass market audio synthesis, home computing, and a whole new way of making music.Â
The important thing to remember is that technology didnât make the music happen. People did, by adapting and responding to the moment they lived in.Â
âOne of the challenges we face being in the present is the inability to see it as a transition,â says patten. âWhen we describe what it is to be a musician, it's within a really specific temporal field, when things are a certain way.â Music-making technologies âcome and go. Like the electric guitar, the CD, turntables. All of these things create and carve out circumstances and behaviors that are very real, but they're never static. We should consider that the way things are right now isn't necessarily the way that they're always going to be, or the best [way]. So, the death of what we consider now to be a musician: we don't have to view that as a negative.â
Read Part 1 of this article.
Text: Angus Finlayson
Images: Veronika Marxer
Have you tried making music with AI tools? Share your results and experience with the Loop Community on Discord.